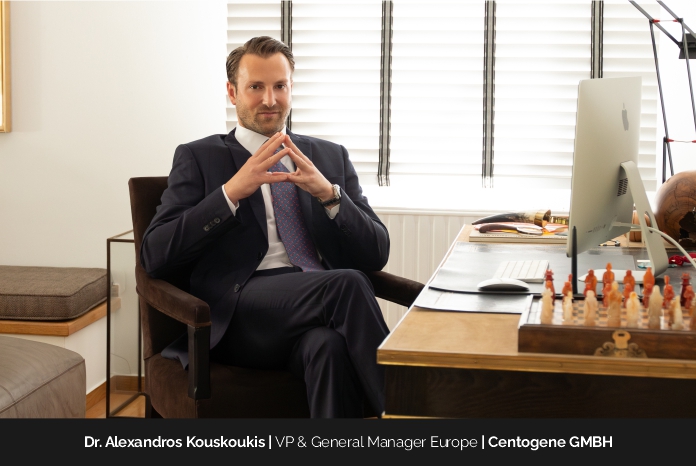
The world today is evolving rapidly and Artificial Intelligence (AI) is playing a crucial role in this acceleration in almost every sector. Healthcare industry is one of the primary ones and will gain significant benefits with the application of AI. It will allow us to use the existing data to make safer and more accurate decisions which will lead to new drugs development, better and faster diagnosis, as well as to new gene therapies. Understanding the complexity and variety of datasets is key to feed the appropriate data to the AI platforms. AI applications are attracting huge investments worldwide from venture funds and tech giants in order to leverage high performance computing and vast datasets to accelerate breakthroughs in complex disease treatment. AI is leading the way during the fourth industrial revolution aside with other technologies like internet of things, blockchain, robotics and automation, 5G and quantum computing.
Large language models (LLMs) have been extensively used in translating things or creating text based on a specific subject. It has been a huge success for AI and the reason is the abundance of existing data. Every email or any kind of script can theoretically be used to improve the models and make them more accurate in creating content or predicting an answer. On the contrary, the data needed to feed the AI models in healthcare are quite limited, creating an imminent need to produce vast amounts of data. High throughput systems have been implemented more and more over the course of the years in order to address the ongoing needs of AI systems. Especially in genetics, with the cost decrease in equipment and automation, laboratories have acquired higher throughput capabilities in order to address the massive need for more data. The new trend is multiomics in order to combine information from various types of molecules like DNA, RNA, enzymes, proteins to study their interactions and build a more comprehensive picture of the disease.
AI in genetics addresses the need to analyze and interpret the genetic data in order to support research findings, accurate diagnosis and treatment selection or even development. Specifically, AI is aiding the processing and analysis of large scale genome sequencing data in an efficient way. The proper identification of variants (variant calling), their annotation with good quality scores and the variant prioritization are crucial steps in this process. Adding additional layers of omics like transcriptomics, proteomics or enzyme testing helps us create more complete datasets to feed the machine learning algorithms. Billions of dollars have been spent over the years in Minimal Residual Disease (MRD) and Multicancer Early Detection (MCED) programs showing promising results in cancer. The improvements will rely on the production of more and diversified data. A recent Nature paper addressed the clinical impact of the 100.000 genomes project in UK and the outcome was that only a few patients followed the therapeutic recommendations. This highlights the complexity of these projects and the need for more datasets which will allow the proper variant identification and with these high quality data the AI based drug discovery. Currently the amount of biological data is very limited, but serious attempts are being undertaken to innovate in scaling biological data generation.
Disease risk prediction is another very important area where AI algorithms analyze genetic data to predict an individual’s risk of developing a disease. Combined with lifestyle data, electronic health records and wearable device data it provides a more complete picture of the risks an individual is having to develop a disease like cancer, diabetes, cardiovascular, neurodegenerative and infectious diseases, as well as rare genetic disorders. It enables early intervention, personalized healthcare and better resource allocation in medical practice. The machine learning algorithms used, need to incorporate data constantly in order to improve over time, ensuring that predictions are more accurate and relevant.
Personalized medicine is one of the milestones that AI will have one of the biggest effects in the future. AI will enable personalized medicine by identifying the way a patient’s unique genetic profile is influencing the response to treatments. Key applications include genomic analysis, polygenic risk scores (PRS), drug development and repurposing, treatment optimization, pharmacogenomics and real time monitoring. The main technologies used are machine and deep learning to identify correlations and patterns in the datasets, natural language processing which extracts insights from unstructured clinical data and reinforcement learning that is learning from outcomes over time, particularly in complex and dynamic conditions like cancer.
Another very promising area where AI is expected to have a huge impact is gene editing and synthetic biology through CRISPR optimization and synthetic gene design. CRISPR based gene editing will be optimized by AI models by predicting the most effective and error free sites in order to improve the outcome. Specifically, AI will improve the guide RNA (gRNA) design optimization in order to navigate the enzyme Cas9 more accurately to the target site and predict the repair mechanisms outcomes to achieve better results. In synthetic gene design, AI will allow the creation of synthetic genes and biological systems to be used in research and in therapy testing. The AI design of synthetic genes and pathways will ameliorate the gene circuits and therefore, the efficiency of the engineered biological systems. Other key benefits entail the accelerated research and development, cost reduction and enhanced therapeutic applications.
Last but not least, the ethical and social implications of the use of AI in genetics is an issue that concerns everybody around the world. The handling of the data needs to be responsible, ensuring privacy and compliance to the regulations like GDPR. AI algorithms need to be unbiased in order to avoid unequal healthcare outcomes which will ensure that healthcare professionals can trust the AI predictions and lead to general adoption. Governmental and regulatory bodies like FDA must continuously monitor the developments in AI in order for rigorous validation to be implemented.